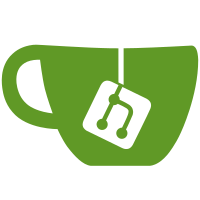
The nixpkgs-unstable channel's programs.sqlite was used to identify packages producing exactly one binary, and these automatically added to their package definitions wherever possible.
84 lines
2.2 KiB
Nix
84 lines
2.2 KiB
Nix
{ lib
|
|
, stdenv
|
|
, python
|
|
, fetchurl
|
|
, anki
|
|
}:
|
|
|
|
python.pkgs.buildPythonApplication rec {
|
|
pname = "mnemosyne";
|
|
version = "2.10.1";
|
|
|
|
src = fetchurl {
|
|
url = "mirror://sourceforge/project/mnemosyne-proj/mnemosyne/mnemosyne-${version}/Mnemosyne-${version}.tar.gz";
|
|
sha256 = "sha256-zI79iuRXb5S0Y87KfdG+HKc0XVNQOAcBR7Zt/OdaBP4=";
|
|
};
|
|
|
|
nativeBuildInputs = with python.pkgs; [ pyqtwebengine.wrapQtAppsHook ];
|
|
|
|
buildInputs = [ anki ];
|
|
|
|
propagatedBuildInputs = with python.pkgs; [
|
|
cheroot
|
|
cherrypy
|
|
googletrans
|
|
gtts
|
|
matplotlib
|
|
pyopengl
|
|
pyqt6
|
|
pyqt6-webengine
|
|
argon2-cffi
|
|
webob
|
|
];
|
|
|
|
prePatch = ''
|
|
substituteInPlace setup.py \
|
|
--replace '("", ["/usr/local/bin/mplayer"])' ""
|
|
'';
|
|
|
|
# No tests/ directory in tarball
|
|
doCheck = false;
|
|
|
|
postInstall = ''
|
|
mkdir -p $out/share/applications
|
|
mv mnemosyne.desktop $out/share/applications
|
|
'';
|
|
|
|
dontWrapQtApps = true;
|
|
|
|
makeWrapperArgs = [
|
|
"\${qtWrapperArgs[@]}"
|
|
];
|
|
|
|
meta = {
|
|
homepage = "https://mnemosyne-proj.org/";
|
|
description = "Spaced-repetition software";
|
|
mainProgram = "mnemosyne";
|
|
longDescription = ''
|
|
The Mnemosyne Project has two aspects:
|
|
|
|
* It's a free flash-card tool which optimizes your learning process.
|
|
* It's a research project into the nature of long-term memory.
|
|
|
|
We strive to provide a clear, uncluttered piece of software, easy to use
|
|
and to understand for newbies, but still infinitely customisable through
|
|
plugins and scripts for power users.
|
|
|
|
## Efficient learning
|
|
|
|
Mnemosyne uses a sophisticated algorithm to schedule the best time for
|
|
a card to come up for review. Difficult cards that you tend to forget
|
|
quickly will be scheduled more often, while Mnemosyne won't waste your
|
|
time on things you remember well.
|
|
|
|
## Memory research
|
|
|
|
If you want, anonymous statistics on your learning process can be
|
|
uploaded to a central server for analysis. This data will be valuable to
|
|
study the behaviour of our memory over a very long time period. The
|
|
results will be used to improve the scheduling algorithms behind the
|
|
software even further.
|
|
'';
|
|
};
|
|
}
|